Introduction
Determining the appropriate number of nurses to staff hospital wards remains a critical challenge for healthcare administrators worldwide. Efficient and effective nurse staffing directly impacts both the financial stability of hospitals and, more importantly, the quality and safety of patient care. Understaffing can lead to increased risks of adverse patient outcomes and burnout among nursing staff, while overstaffing can strain hospital budgets. Therefore, the quest for reliable tools to accurately assess nurse staffing requirements is paramount.
Numerous patient classification systems and tools have been developed to aid in this complex process. Among these, the Safer Nursing Care Tool (SNCT) stands out as a widely adopted system within the UK’s National Health Service (NHS). Endorsed by the National Institute for Health and Care Excellence (NICE), the SNCT aims to provide a structured approach to estimating the nursing staff needed based on patient acuity and dependency. In essence, the SNCT functions as a foundational element for what could be described as a “Safer Nursing Care Tool Calculator”—a system designed to quantify staffing needs and promote safer patient care environments.
While the SNCT’s prevalence is undeniable, rigorous, independent evaluations of its effectiveness and precision have been notably scarce. This study addresses this critical gap by delving into two key aspects of the SNCT’s performance. First, it investigates the statistical precision of staffing estimates derived from the SNCT, exploring how sample size influences the reliability of these estimates. Understanding the precision is crucial for hospital administrators who rely on such tools to make informed staffing decisions. Is a 20-day sample, as recommended, truly sufficient? Can a “safer nursing care tool calculator” built upon the SNCT provide consistently accurate numbers with limited data?
Second, and perhaps more importantly, this research examines the extent to which staffing levels recommended by the SNCT align with the professional judgment of nurses themselves. Do the numbers generated by a “safer nursing care tool calculator” truly reflect the on-the-ground realities of nursing workload and staffing adequacy? By comparing SNCT-estimated staffing requirements with nurses’ assessments of whether they have “enough staff for quality care,” this study aims to validate the SNCT against the gold standard of professional nursing expertise. Furthermore, the study explores whether factors beyond patient classification, such as unit specialty and hospital layout, influence staffing adequacy, considerations that a basic “safer nursing care tool calculator” might overlook.
This observational study provides crucial evidence about the reliability and validity of the SNCT as a “safer nursing care tool calculator” component. By understanding its strengths and limitations, hospitals can better leverage the SNCT, or similar tools, to optimize nurse staffing, enhance patient safety, and ultimately improve the quality of care delivery.
Methods and Materials
This research draws upon a comprehensive dataset collected over a year (2017) across 81 acute medical/surgical units (encompassing 2178 beds) within four NHS hospital trusts in England. The methodology and data collection are detailed in a previous publication in the NIHR Journals Library Health Services and Delivery Journal. The study employed routinely collected data and incorporated nurse-reported assessments to link staffing levels, SNCT ratings, and professional judgments of staffing adequacy.
Setting and Participants:
The study encompassed a diverse range of hospital settings, including a university teaching hospital, two general hospitals, and a specialist cancer hospital (with two sites). These hospitals served varied populations, including rural communities, inner-city areas with socioeconomic challenges, and national referral centers for specialized care. All participating hospitals routinely reviewed their nurse staffing establishments at least biannually. Notably, two hospitals had established SNCT usage prior to the study, while the other two adopted the tool shortly before the research commenced, offering a spectrum of SNCT implementation experience.
The study focused on general medical and surgical units providing 24-hour inpatient care. Units outside the SNCT’s scope, such as pediatrics, intensive care, maternity, neonatal, and palliative care, were excluded. Additionally, units with highly specialized staffing needs, like bone marrow transplant and isolation units, were also excluded based on local co-investigator assessments. The final unit sample represented a significant 74% of all beds across the four participating hospitals, providing a robust representation of general acute care settings.
Data Sources and Measures:
Data collection involved shift leaders (nurses in charge of a shift) who were trained in SNCT utilization and staffing adequacy assessments. These shift leaders, at least twice daily, recorded patient classifications within the SNCT categories and provided judgments on staffing adequacy using a microsurvey. Data entry was facilitated through electronic systems, with laminated sheets providing supporting information and guidance readily accessible near unit computers. Further data, including electronic rosters and patient administration system records (admissions, discharges), were routinely collected for administrative purposes. Each hospital also provided unit profiles detailing the main specialty and layout, including bed numbers and single-room proportions.
Study Variables:
The study utilized the most current SNCT multipliers available at the study’s outset. Patient counts in each SNCT category, as reported by shift leaders, were used to calculate a weighted average multiplier per unit and day. This multiplier was then applied to the patient count from the patient administration system to estimate the required unit establishment (number of nurses to employ). Morning assessments (or later assessments if morning data were missing) and patient counts at 07:00 served as the basis for the primary analysis. The SNCT calculation yields the staffing establishment, inclusive of allowances for staff leave and sickness. To derive the implied daily hours of staff time, a 37.5-hour working week for a full-time equivalent was assumed, and the 22% ‘uplift’ (for leave and sickness) inherent in the SNCT establishment was removed.
To account for skill mix, the average observed skill mix on each unit was used as a proxy for the planned registered nurse to nursing assistant ratio. Patients requiring one-to-one supervision (‘specialing’), while not explicitly addressed in the SNCT, were accounted for by adding their required hours to the estimated staffing requirement for days where such patients were present. However, when estimating establishments for employment purposes, no additional allowance was made for ‘specialing’, as this was considered to be implicitly included within the average care observed in the SNCT multiplier development.
Staffing levels were measured using electronic roster data, capturing hours worked by registered nurses and nursing assistants daily (07:00 to 07:00). These hours were then divided by patient days to calculate hours per patient day (HPPD) for each unit and day. Staffing shortfall was quantified by subtracting the SNCT-estimated required hours (plus specialing requirements) from the actual deployed hours. Negative shortfalls indicated staffing levels exceeding the estimated requirement. Daily patient turnover per staff member was also calculated (patient entries and exits divided by total staff hours).
Nurse-reported outcomes focused on staffing adequacy, captured through a microsurvey embedded in the daily SNCT assessments (Box 1). This microsurvey included three items, chosen for pragmatic reasons to minimize administrative burden while capturing key aspects of staffing adequacy:
Box 1. Staffing adequacy questions.
Questions:
- Were there enough nursing staff to provide quality care on the last shift?
- Was necessary nursing care left undone (missed) on the last shift because there were too few nursing staff?
- Were staff breaks missed on the last shift because there were too few nursing staff?
Data Cleaning and Analysis:
Data cleaning, processing, and statistical analyses were performed using R statistical software. Extreme values for staffing shortfall (outside mean ±3 SD) were removed to eliminate atypical periods or data entry errors, affecting approximately 1.5% of cases. Units undergoing significant changes (moves, patient population shifts, bed number alterations) were treated as separate units. Reverse coding errors in staffing adequacy questions, identified in some units of one hospital, were corrected using logical rules, with sensitivity analyses conducted to assess the impact of excluding this hospital entirely.
To assess the accuracy of the SNCT-based establishment estimates, bootstrap resampling (1000 samples) was used to estimate mean establishment and 95% confidence intervals (CIs) for each unit, starting with the recommended 20-day sample size and increasing the sample size incrementally. Precision was calculated as half the CI width as a percentage of the mean, and the absolute CI width in whole-time equivalent (WTE) staff members was also determined. The number of units achieving CI widths of ≤2 WTE and ≤1 WTE was calculated.
Multilevel logistic regression models were used to analyze the relationship between staffing deficits (HPPD) and nurse-reported staffing adequacy measures. These models, fitted using the glmer function from the lme4 package in R, accounted for the hierarchical data structure (staffing nested within unit, unit nested within hospital). Control variables included day of the week, proportion of single rooms, turnover, and unit specialty (surgical vs. medical/mixed). Models examined the association of staffing adequacy outcomes with deviations in both registered nurse and nursing assistant staffing from SNCT-estimated requirements, as well as total hour deviations and skill mix (registered nurse proportion).
Non-linear relationships and interactions between staffing variables and other factors were explored by introducing quadratic terms and interaction effects, with model fit assessed using Akaike information criteria (AIC) and Bayesian information criteria (BIC).
Patient and Public Involvement:
Patient and public involvement was considered throughout the research process, guided by experts in the field. While direct patient/public involvement in question prioritization was deemed less relevant due to the technical nature of the study, a lay member with expertise in safe staffing and patient involvement in healthcare guideline development was included in the steering group. Ward-based staff nurses were considered as key end-users of the research, and engagement with this group was prioritized through social media and consultation events.
Ethical Approval and Registration:
The study was prospectively registered and received ethical approval from the Health Research Authority and the University of Southampton Ethics committee. Patient data were pseudoanonymized at source, and no direct patient data collection occurred, negating the need for NHS Research Ethics Committee approval.
Results
The study achieved high data completeness, with SNCT ratings recorded on 96% of occasions and staffing adequacy question responses on 85% or more of occasions. After data cleaning and linkage, the analysis included over 22,000 unit days for each staffing adequacy outcome measure.
Significant variation in average unit staffing levels and skill mix was observed across hospitals and units within hospitals (Table 1). At the hospital level, average estimated staffing requirements generally aligned with observed staffing levels in three of the four hospitals, although all were slightly understaffed relative to SNCT estimates (by 8% or less). Larger discrepancies between actual staffing and SNCT estimates were evident in smaller, specialized units with more single rooms (apparent overstaffing) and some larger medical units (apparent understaffing). Hospital C, a specialist hospital with numerous small units, exhibited an average unit staffing level 50% higher than the SNCT estimated requirement.
Table 1. Mean and range of units’ average daily staffing levels, skill mix and SNCT estimated staffing requirements
Hospital | Total hours per patient day | Skill mix (% registered nurses) | Estimated staffing requirement |
---|---|---|---|
Mean | Min | Max | |
A | 7 | 5.4 | 10.4 |
B | 6.8 | 5.0 | 8.9 |
C | 10.5 | 7.5 | 14.2 |
D | 6.5 | 5.2 | 8.4 |
All | 7.3 | 5.0 | 8.4 |
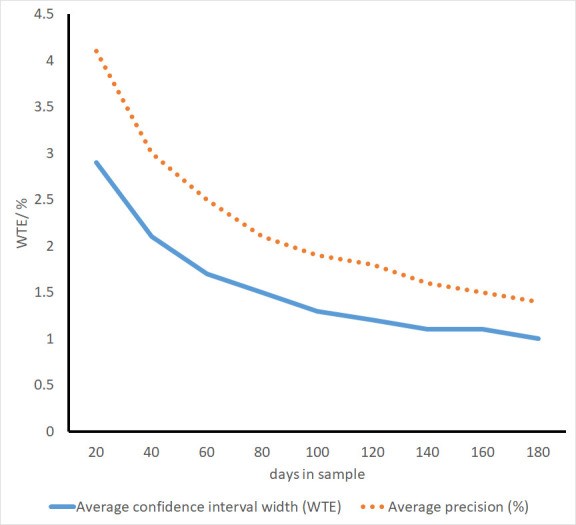
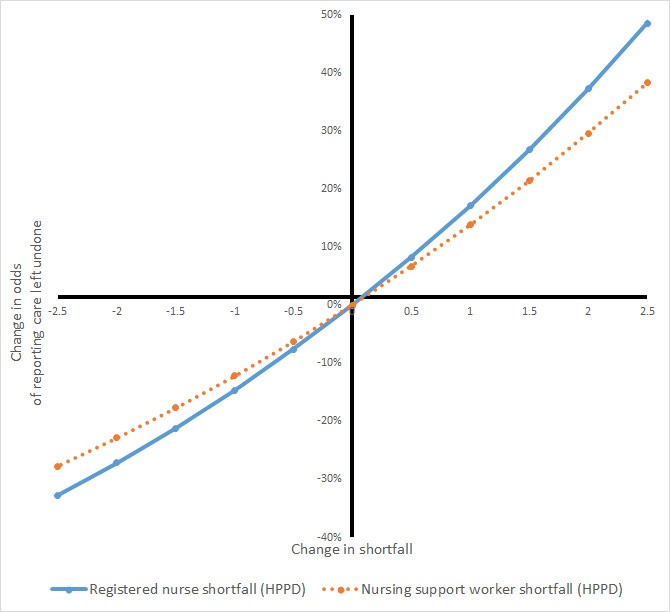
Using the recommended minimum of 20 days’ data, the average precision of SNCT establishment estimates was 4.1%, with unit-level variation ranging from 0.6% to 13.5%. In absolute terms, the average width of the 95% CIs for the establishment was 2.9 WTE staff (approximately mean ±1.5 WTE). Only 27 out of 86 units had a CI width ≤2 WTE, and a mere 3 units had a CI width ≤1 WTE.
Increasing the sample size significantly improved precision (Figure 1). A 40-day sample resulted in CI widths ≤2 WTE for most units (56/86). However, the benefits of larger samples diminished beyond this point. Even with 180-day samples, only 53 out of 77 units achieved a CI width ≤1 WTE (Table 2).
Table 2. Average widths of 95% CIs for the mean using different sample sizes to estimate establishment
Sample size taken for the estimate | Average CI width (WTE) | Average precision (%) | Number units with CI width 1 WTE or less | Number units with CI width 2 WTE or less | Number of units* |
---|---|---|---|---|---|
20 | 2.9 | 4.1 | 3 | 27 | 86 |
40 | 2.1 | 3.0 | 7 | 56 | 86 |
60 | 1.7 | 2.5 | 10 | 64 | 86 |
80 | 1.5 | 2.1 | 20 | 72 | 86 |
100 | 1.3 | 1.9 | 31 | 74 | 82 |
120 | 1.2 | 1.8 | 39 | 74 | 81 |
140 | 1.1 | 1.6 | 44 | 74 | 81 |
160 | 1.1 | 1.5 | 50 | 75 | 80 |
180 | 1.0 | 1.4 | 53 | 73 | 77 |
Across all units, nurses in charge reported “enough staff for quality care” on an average of 78% of shifts (unit range 24%-100%). “Necessary nursing care left undone” due to understaffing was reported on 5% of shifts (range 0%-25%), and “missed breaks” on 5% of shifts (range 0%-29%).
Staffing shortfalls relative to SNCT-estimated requirements were significantly associated with nurses’ perceptions of staffing adequacy (Table 3). In multivariable models, each registered nurse hour shortfall was associated with an 11% reduction in the odds of nurses reporting “enough staff for quality,” a 14% increase in the odds of reporting “nursing care left undone,” and a 12% increase in the odds of “staff missing breaks.” Similar findings were observed for nursing assistant shortfalls.
Table 3. Association between staffing shortfall and nurse perceptions of staffing adequacy: univariable and multivariable models
| Variable | Enough staff for quality | Nursing care left undone | Staff breaks missed |
|—|—|—|—|—|—|—|—|—|—|—|—|
| | OR* | Adjusted OR | 95% CI | P value | OR* | Adjusted OR | 95% CI | P value | OR* | Adjusted OR | 95% CI | P value |
| Registered nurse shortfall (HPPD) | 0.94 | 0.89 | (0.87 to 0.92) | 0.000 | 1.09 | 1.14 | (1.08 to 1.20) | 0.000 | 1.08 | 1.12 | (1.06 to 1.18) | 0.000 |
| Nursing assistant shortfall (HPPD) | 0.90 | 0.86 | (0.83 to 0.89) | 0.000 | 1.08 | 1.14 | (1.07 to 1.20) | 0.000 | 1.06 | 1.11 | (1.05 to 1.17) | 0.000 |
| Turnover (per nursing hour) | 0.35 | 0.91 | (0.30 to 2.75) | 0.863 | 7.16 | 3.36 | (0.60 to 18.72) | 0.167 | 3.85 | 4.94 | (0.95 to 25.78) | 0.058 |
| Unit type | | | | | | | | | | | | |
| Medical or mixed (ref) | 1.00 | 1.00 | | | 1.00 | 1.00 | | | 1.00 | 1.00 | | |
| Surgical | 0.57 | 0.54 | (0.31 to 0.92) | 0.023 | 2.00 | 2.13 | (1.19 to 3.82) | 0.011 | 2.13 | 2.15 | (1.23 to 3.78) | 0.008 |
| Proportion single rooms | 0.89 | 0.54 | (0.18 to 1.66) | 0.283 | 1.58 | 3.01 | (0.93 to 9.71) | 0.065 | 1.05 | 2.06 | (0.65 to 6.56) | 0.221 |
| Day of week | | | | | | | | | | | | |
| Monday (ref) | 1.00 | 1.00 | | | 1.00 | 1.00 | | | 1.00 | 1.00 | | |
| Tuesday | 1.11 | 1.12 | (0.99 to 1.26) | 0.079 | 0.86 | 0.86 | (0.69 to 1.06) | 0.160 | 0.94 | 0.71 | (0.58 to 0.88) | 0.001 |
| Wednesday | 1.28 | 1.28 | (1.13 to 1.45) | 0.000 | 0.95 | 0.96 | (0.78 to 1.18) | 0.684 | 1.01 | 0.61 | (0.49 to 0.76) | 0.000 |
| Thursday | 1.09 | 1.08 | (0.96 to 1.23) | 0.200 | 0.90 | 0.91 | (0.73 to 1.13) | 0.383 | 0.96 | 0.81 | (0.66 to 1.00) | 0.045 |
| Friday | 1.03 | 1.03 | (0.91 to 1.17) | 0.610 | 0.93 | 0.93 | (0.74 to 1.15) | 0.488 | 1.03 | 0.79 | (0.64 to 0.97) | 0.028 |
| Saturday | 1.28 | 1.29 | (1.14 to 1.47) | 0.000 | 0.74 | 0.75 | (0.60 to 0.95) | 0.016 | 0.86 | 0.50 | (0.40 to 0.64) | 0.000 |
| Sunday | 1.02 | 1.02 | (0.90 to 1.15) | 0.811 | 1.08 | 1.11 | (0.90 to 1.37) | 0.310 | 0.84 | 0.82 | (0.66 to 1.01) | 0.058 |
| Variance partition coefficient for units** | | 0.22 | | | | 0.22 | | | | 0.23 | | |
| Variance partition coefficient for hospitals** | | 0.12 | | | | 0.17 | | | | 0.11 | | |
| Akaike information criterion | | 20 697 | | | | 8377 | | | | 8094 | | |
| Bayesian information criterion | | 20 809 | | | | 8490 | | | | 8206 | | |
Surgical units were associated with lower odds of nurses perceiving adequate staffing compared to medical or mixed units, with 46% lower odds of reporting “enough staff for quality” and higher odds of reporting “care left undone” or “missed breaks.” Units with a higher proportion of single rooms also showed lower odds of reported staffing adequacy, although these relationships were not statistically significant and CIs were wide. Similarly, units with higher turnover showed increased odds of reporting “care left undone” and “missed breaks,” again with wide CIs and non-significant relationships. Saturday shifts were associated with higher odds of reported staffing adequacy compared to Mondays.
Tests for non-linear relationships revealed a significant non-linear term for registered nurse staffing in predicting “nursing care left undone,” but no clear indication of improved model fit or a threshold effect. Figure 2 illustrates the essentially linear relationship observed, with no apparent staffing level at which the likelihood of care left undone plateaus. Non-linear terms were not significant for other outcomes and worsened model fit. Interaction effects between staffing shortfalls and other variables were also non-significant and decreased model parsimony. Models using overall care hour shortfall (combined registered nurse and assistant hours) yielded similar results, with no significant skill mix effects. Sensitivity analyses excluding data from the hospital with coding errors did not substantially alter the findings.
Discussion
This study provides the first independent evaluation of the SNCT, a widely used tool in English hospitals to determine nurse staffing levels. While the SNCT, functioning as a “safer nursing care tool calculator,” offers a structured approach, our findings reveal important considerations regarding its precision and validity.
Using the recommended minimum 20-day sample, estimates of required nurse staffing establishments exhibited an average precision of 4.1%. However, this average masked considerable unit-level variation and substantial confidence intervals for absolute staff numbers. For many units, the potential variation from the “true” staffing requirement exceeded two full-time equivalent staff members. While a 4.1% average precision might seem superficially acceptable for a “safer nursing care tool calculator,” the practical implications of such variability in real-world staffing decisions are significant.
Increasing the data collection period to 40 days substantially improved precision, bringing estimates within ±1 staff member for most wards. However, gains diminished with larger samples, and even with 180 days of data, achieving a CI width of no more than one staff member remained elusive for a significant proportion of units. This suggests that relying solely on a “safer nursing care tool calculator” based on limited data may lead to imprecise staffing decisions.
The study’s findings underscore that while the SNCT provides a valuable measure of nursing workload, factors beyond patient classification significantly influence staffing adequacy. Nurses consistently reported lower staffing adequacy on surgical units compared to medical or mixed units, even when accounting for SNCT-estimated needs. This suggests that surgical settings may have inherent workload demands not fully captured by the SNCT’s patient acuity classifications. Similarly, units with higher proportions of single rooms and higher patient turnover were associated with lower perceived staffing adequacy, pointing to the increased indirect care demands and surveillance needs in such environments. These factors highlight the limitations of a basic “safer nursing care tool calculator” that solely relies on patient classification data.
Crucially, the study found a linear relationship between staffing shortfalls (relative to SNCT estimates) and negative nurse-reported outcomes (insufficient staff, care left undone, missed breaks). No evidence of a threshold effect emerged, suggesting that even staffing levels meeting SNCT recommendations may not be perceived as truly “optimal” by nurses. This challenges the notion that a “safer nursing care tool calculator” alone can define optimal staffing, as continuous improvement in staffing levels appears to correlate with better perceived adequacy and reduced missed care.
The similar, independent effects of registered nurse and nursing assistant shortfalls emphasize the distinct yet complementary roles of both staff groups in ensuring patient safety and quality care. While a “safer nursing care tool calculator” might provide overall staffing numbers, the nuanced skill mix and specific contributions of RNs and nursing assistants must be carefully considered, as they are not simply interchangeable.
Limitations:
The study acknowledges several limitations. While training was provided for SNCT use, the study’s scale may have resulted in less precise SNCT ratings compared to expert assessments. However, the observed variability in precision is unlikely solely attributable to this factor. The study’s real-world setting reflects routine SNCT application, where daily assessments by shift leaders are increasingly common. Coding errors in staffing adequacy assessments were detected in one hospital, potentially indicating less systematic errors elsewhere, which could attenuate the estimated relationships. The observational design limits causal inferences, and the reliance on subjective nurse reports, while validated against patient outcomes in other studies, introduces a subjective element. The study’s sample, while large, was drawn from only four hospitals in England, potentially limiting generalizability. Finally, the study did not explore the consequences of overstaffing.
Conclusion
This study provides critical insights into the SNCT as a “safer nursing care tool calculator” component. It demonstrates that while the SNCT can provide a reliable estimate of unit staffing establishment, achieving precise estimates, particularly within one WTE staff member, often requires larger samples than the recommended minimum. Furthermore, factors beyond patient classification, such as unit specialty and layout, significantly influence perceived staffing adequacy and should be considered alongside SNCT outputs.
The linear relationship between staffing shortfalls and negative nurse-reported outcomes challenges the notion of a fixed “optimal” staffing level defined solely by a “safer nursing care tool calculator.” Instead, continuous improvement in staffing appears beneficial. The study reinforces that professional judgment remains paramount in nurse staffing decisions. A “safer nursing care tool calculator,” based on tools like the SNCT, should be viewed as a valuable adjunct to, not a replacement for, the expertise and insights of nurses on the front lines of patient care. Future research should explore tailoring “safer nursing care tool calculator” methodologies to specific unit types and incorporating dynamic factors like patient turnover and unit layout to enhance their accuracy and relevance in diverse hospital settings.
References
(Keep original references – same as provided in the original article)
Associated Data
(Keep original associated data links – same as provided in the original article)